In order to overcome systematic racial biases that can show up in algorithms, three scholars at a June 19 Brookings Institution webinar suggested putting recognition of historical racial bias at the center of algorithm development from the very beginning of the design process.
Because of systematic racism that permeates all fields, including tech, Fay Cobb Payton, IT and business analytics professor at North Carolina State University, explained that scientists creating the algorithms may not be addressing the right issues from the start of the design process. That kind of misstep excludes certain populations from the algorithm’s consideration, she said.
“The exclusion of the populations in the algorithmic design process from the beginning holds implications for the science in the end,” Cobb Payton explained. For example, if an organization is using an algorithm to track who it serves, “when you don’t factor in race, you get a totally different picture of who actually gets services and how many services they will get,” she added.
Rashawn Ray, governance studies fellow at Brookings, acknowledged the misconception that algorithms are free of bias because of their roots in math and science. “They are created by people,” he said. “What goes into the algorithm informs what we get out of it,” Ray added.
Often, algorithms are approached in what Ray called a “colorblind” way. Race isn’t given the proper weight as a factor to consider when designing algorithms, which hurts the tech community’s abilities to properly address the systematic inequalities, Ray said.
“In order to fundamentally deal with racism in the algorithms we have, we have to center race in the models that we create,” Ray asserted.
Director of the Economic Justice Project Dariely Rodriguez further explained how these systematic inequalities are furthered by algorithms. “Oftentimes, what the algorithms can do is replicate historic biases and historic discrimination,” she said. For example, the tech industry historically lacks employees of color, so relying on past data to make future decisions continues that pattern of exclusion, she said.
To begin to correct these inequalities, Cobb Payton and Ray both recommended a closer analysis of the training data and early algorithmic development. Algorithmic fairness should be built in from the start, and the source and cleanliness of training data must be evaluated, Cobb Payton said. If the model’s outcomes have patterns of inequity or other adverse effects, Ray added that “small data” can be examined to explain and resolve the discrepancies.
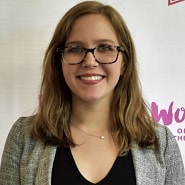