A new study by the National Institute of Standards and Technology (NIST) revealed that data, algorithms, and application processes are the biggest determinants of facial recognition software’s ability to identify sex, age, and racial background.
The December 2019 report, intended to inform Federal policymakers and software developers, discovered that most face recognition algorithms struggle to match two images of the same person from one demographic group to another. NIST called these “demographic differentials.”
Researchers evaluated 189 algorithms and 99 developers from industry and academia. They tested the software’s ability to confirm a photo matches a different photo of the same person – called one-to-one matching – and whether the software could detect if a person in a photo has a database match – called one-to-many matching.
“In a one-to-one search, a false negative might be merely an inconvenience — you can’t get into your phone, but the issue can usually be remediated by a second attempt…But a false positive in a one-to-many search puts an incorrect match on a list of candidates that warrant further scrutiny,” Patrick Grother, the report’s main author, explained in a press release.
While Grother added that the conclusions in the study mostly applied to individual programs, he highlighted five key findings:
- Higher rates of false positive for one-to-one matches of Asian and African-American faces compared to images of Caucasians;
- High rates of false positives for one-to-one matches of Asians, African-American, and native populations among U.S. algorithms;
- No difference in false positives for one-to-one matches of Asian and Caucasian faces for algorithms developed in Asia;
- Higher rates of false positive for one-to-many matches of African-American women compared to other populations; and
- Not all algorithms shared the same rates of false positives for one-to-many matches across demographic groups.
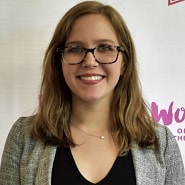