In the age of machine learning, there’s a fine line between collecting enough employee data for insider threat programs and ensuring personal privacy, a line that Americans may have to culturally define in the near future, according to experts speaking at an Intelligence and National Security Alliance (INSA) event on Monday.
“Are we willing to sacrifice some of the civil liberties and some of those privacy issues in order to create that capability? So that’s a policy discussion, it’s not a capability discussion. We have the capability right now,” said Michael Seage, director of the Defense Insider Threat Management and Analysis Center. “I honestly think that Congress is going to have to sit down and give this a good, thorough think. They’re going to have to balance that tension between civil liberties and privacy and the effectiveness of the insider threat program. And we honestly have to ask ourselves as a culture how far we want to go down that road, what are we willing to sacrifice for security.”
According to Seage, many people in the defense sector are hesitant to encroach any further on privacy protections because they risk looking like Big Brother.
But Wayne Belk, Co-Director of the National Counterintelligence and Security Center’s National Insider Threat Task Force, said while it’s very hard to get past the worry that insider threat programs are like Big Brother, the government has standards for how that data is used and who can access it.
“There are specific requirements in the minimum standards that address that issue,” said Belk, explaining that those handling the data must meet specific training requirements beforehand.
According to a report prepared by INSA and released on Monday, organizations can gain great insight into the potential of an insider becoming a threat by monitoring personality traits, stressors, and significant life events of employees through machine learning.
Vinny Corsi, threat and fraud manager at IBM and a member of the INSA subcommittee that prepared the research explained that the words and phrasing people use on a daily basis can reveal personality traits like narcissism or altruism, and machines can be trained to interpret those things. Machines can also survey the text of communications to extrapolate the likelihood of a stressful emotion or a significant life event occurring for a particular employee.
Corsi explained that sometimes these words are obvious, like “rings” and “love” being associated with a recent marriage. But the associations that aren’t obvious to people, like “home” and “morning” referring to recent travel, can be picked up by trained machines.
“This shows you why you need machine learning: some events aren’t obvious,” said Corsi.
However, Corsi argued in favor of placing strong restrictions on who and what organization is allowed to access that data and how it is labeled.
“Not everybody needs to see the results,” said Corsi, adding that it’s best to strip identities from the data as often as possible, especially when an organization outsources their insider threat monitoring to another company. “What you can really do is anonymize the data.”
Bryan Denson, DOD and intelligence programs account manager at TransUnion, said that consent and permissible use of personal data should be an important legal discussion for insider threat programs.
“Discuss those upfront, early, and often,” said Denson.
Dan McGarvey, senior principal business analyst at Alion Science and Technology and also a member of the subcommittee that compiled the research, added that there should be an insider threat working group who is responsible for considering personal privacy and recording how the data is collected, stored, and maintained.
“If you do all of that, I think you can ensure that you have a pretty good grasp on the security aspects,” said McGarvey.
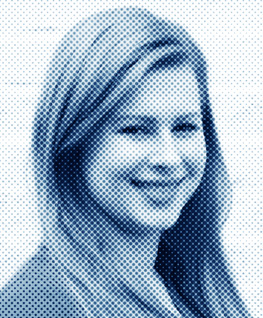