While facial recognition technologies offer huge opportunities to Federal agencies, overcoming algorithmic biases with the help of diverse data sets is essential for full deployment, industry experts said Oct. 29 at General Dynamics Information Technology’s Women + Technology event produced in association with MeriTalk.
The panelists – Catherine Tilton, Chief Technologist for Biometrics at GDIT, Janice Kephart, CEO of Identity Strategy Partners, and Dr. Sumeet Dua, associate vice president for Research and Partnerships at Louisiana Tech University – convened to cover strategies for mitigating bias and improving AI decision making.
One of the major causes of biases within facial recognition is a “lack of diversity in training materials,” Dua said. However, he did note that there “are efforts underway to address this underpinning concern.”
The United States faces a unique challenge in overcoming algorithmic biases, Kephart explained. “Something that occurs in the United States is a high emphasis on privacy,” she said. Because the U.S. considers facial data to be private, data sets must come from publicly available data.
Who doesn’t expect facial privacy, Kephart asked, saying the answer is Hollywood. She explained this means that data sets used to train AI algorithms are dominated by images of white people. “Our protection of PII [personally identifiable information] makes it difficult for us to achieve accuracy of face images.”
Citing a recent National Institute of Standards and Technology report, Tilton explained that “in general the error rates are higher for women than for men, and higher for non-whites than for white.” However, she further noted that “this also varies highly by vendor.” For Tilton, this variance between vendors is a positive thing “that indicates to me that it is possible to drive out this behavior, and it indicates just how important vendor selection is.”
Driving home the point Kephart made, Tilton argued that “the availability of sufficient amounts of accurately labeled data is critical” to overcoming error rates between demographic groups. Large, diverse data sets are not only important when it comes to training facial recognition technologies, but also to understanding just how accurate and unbiased they are. When measuring false data rates, it’s important to have very large data sets to get a low margin of error, she said.
Tying back to Kephart’s point about privacy, Tilton explained that, unfortunately, the desire to protect an individual’s privacy sometimes leads to disadvantaging the very people that need protection because scientists struggle to secure the datasets they need. In addition to initially securing large diverse datasets, Tilton cited regulations surrounding the use of data as being an obstacle. Namely, requirements to delete data after its immediate purpose is over, the inability to share data with other researchers, and the inability to use social media data.
On top of improving access to data sets, Dua briefly touched on another solution to curbing biases in facial recognition technologies. “There are also algorithmic modifications that can be made” that would halt the biases, Dua said. He noted that researchers are already working to understand what algorithmic changes would need to be made to not only remove biases, but also to improve accuracy.
Removing biases from facial recognition technology is essential to our government’s ability to fully embrace the technology. While there is still a way to address this issue, the panelists seemed confident that the goal of mitigating biases is within reach.
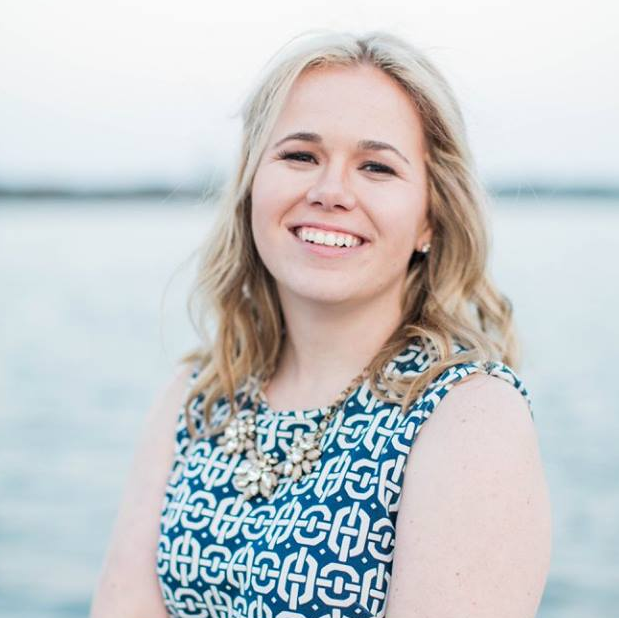